IRD stream
By using the framework of Stream2D
, you can apply functions into
multiple fits files. Here, we try to reduce from raw data to wavelength
calibrated spectra.
Start by setting a path for the directory which contains raw data of ‘Flat’, ‘ThAr’, and ‘Target’ (and ‘Dark’, optionally)
import pathlib
basedir = pathlib.Path('~/pyird/data/20210317/').expanduser()
and some common variables.
band = 'h' #'h' or 'y'
mmf = 'mmf2' #'mmf1' (comb fiber) or 'mmf2' (star fiber)
skipLFC = False #if False, uncertainties are output. mmf1 of y band must be reduced first.
If skipLFC
is False (default), uncertainties and signal-to-noise
ratio at each wavelength are included in output files
(*nw?????_m?.dat* and *ncw?????_m?.dat*), computed by using the
readout noise (RN) in the comb spectrum (in mmf1) of the Y/J band.
Therefore, spectrum of mmf1 in the Y/J band should be reduced first when
skipLFC=False.
If skipLFC=True
, the RN is set by the default value (RN=12
\(e^{-}\)).
Preprocessing of calibration dataset
Set the directory and frame numbers for ‘Flat’ to search for reference apertures for spectrum extraction.
It would be better to use FLAT_COMB data (flat light injected to the LFC fiber) because lights can be found in both fibers so that there is no need to change frame IDs when switching fiber to analyze.
Frame numbers can be set the even numbers. When analyzing H band, they are increased by one by using
fitsid_increment()
.
from pyird.utils import irdstream
## FLAT_COMB
datadir = basedir/'flat/'
anadir = basedir/'reduc/'
flat_comb=irdstream.Stream2D("flat_comb",datadir,anadir)
flat_comb.fitsid=list(range(41704,41804,2))
flat_comb.band=band
print(flat_comb.band,' band')
No fitsid yet.
h band
The function aptrace
searches apertures to be traced.
The number of apertures is specified by
nap
, which should be set 42 (or 21) for H band and 102 (or 51) for YJ band.The apertures begin its search at
cutrow
, which is the row number of the detector (wavelength direction), and continue in the direction of increasing numbers until it matches the appropriate number of apertures. You may as well change the value ofcutrow
if the aperture trace is failed.
# aperture extraction
if band=='h' and flat_comb.fitsid[0]%2==0:
flat_comb.fitsid_increment()
trace_mmf=flat_comb.aptrace(cutrow = 1200,nap=42)
elif band=='y':
trace_mmf=flat_comb.aptrace(cutrow = 1000,nap=102)
median combine:
100%|██████████| 50/50 [00:00<00:00, 106.28it/s]
cross-section: row 1201
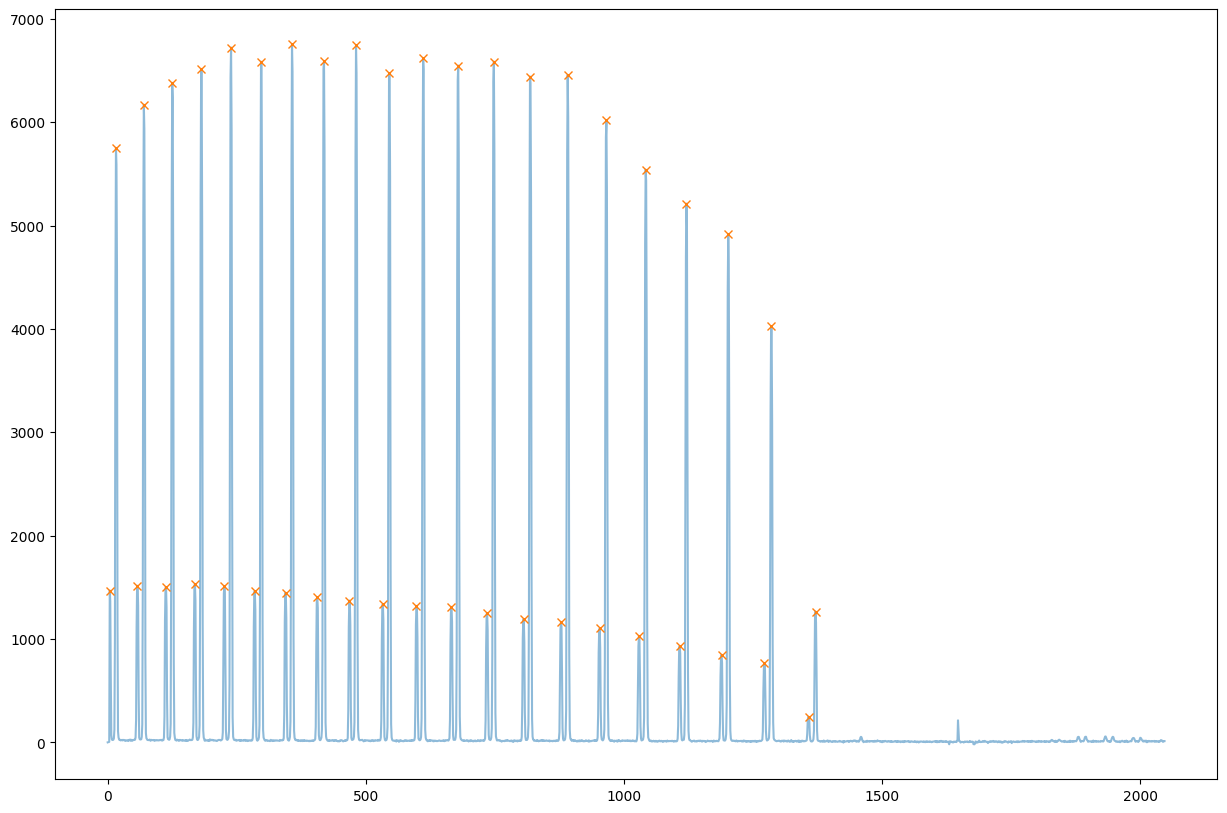
100%|██████████| 42/42 [00:22<00:00, 1.87it/s]
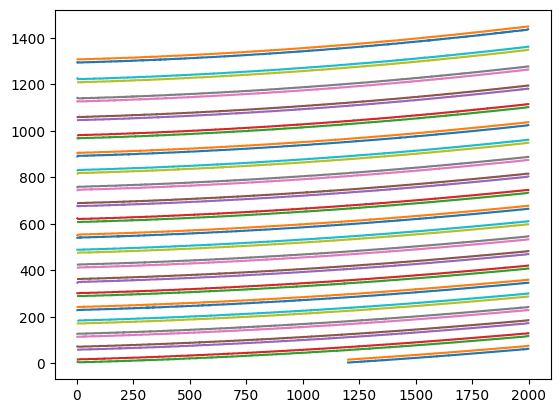
You can change the aperture width if you want. By default, the width is 6 pixels (from -2 to +4) for IRD data and 5 pixels (from -2 to 3) for REACH data.
For example, setting to 6 pixels (from -2 to +4) can be done as follows:
width_str, width_end = 2, 4
trace_mmf.width = [width_str, width_end]
Show trace mask.
trace_mask = trace_mmf.mask()
import matplotlib.pyplot as plt
# apeture mask plot
plt.imshow(trace_mmf.mask())
plt.show()
100%|████████████████████████████████████████████████████████████████████████████████████████████████████████████████| 42/42 [00:00<00:00, 101.46it/s]
100%|████████████████████████████████████████████████████████████████████████████████████████████████████████████████| 42/42 [00:00<00:00, 103.25it/s]
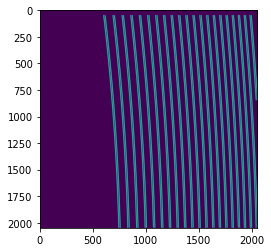
There are two options for the hotpixel mask:
One is made from dark data as follows.
from pyird.image.bias import bias_subtract_image
from pyird.image.hotpix import identify_hotpix_sigclip
## HOTPIXEL MASK:
## DARK
datadir = basedir/'dark/'
anadir = basedir/'reduc/'
dark = irdstream.Stream2D('dark', datadir, anadir,fitsid=[43814]) # Multiple file is ok
if band=='h' and dark.fitsid[0]%2==0:
dark.fitsid_increment()
median_image = dark.immedian()
im_subbias = bias_subtract_image(median_image)
hotpix_mask = identify_hotpix_sigclip(im_subbias)
fitsid: [43814]
median combine:
100%|██████████████████████████████████████████████████████████████████████████████████████████████████████████████████| 1/1 [00:00<00:00, 110.40it/s]
hotpix mask = 0.45 percent
Another option is reading the prepared hotpixel mask as follows.
# example to read a hotpixel mask used in IRD
import pkg_resources
from pyird.io.read_hotpix import read_hotpix
if band=='h':
path=pkg_resources.resource_filename('pyird', 'data/hotpix_mask_h_202210_180s.fits')
elif band=='y':
path=pkg_resources.resource_filename('pyird', 'data/hotpix_mask_y_202210_180s.fits')
hotpix_mask=read_hotpix(path)
Then, select the fiber to analyze. The following operations are just drop even/odd number of apertures from all apertures identified by Flat.
# reduce mmf1 or mmf2
if mmf=='mmf2':
trace_mmf.mmf2() #mmf2 (star fiber)
elif mmf=='mmf1':
trace_mmf.mmf1() #mmf1 (comb fiber)
Wavelength calibration is performed by using reference frames
(Thrium-Argon). We do not need to identify the emission lines by eye;
calibrate_wavelength
automatically refer to the line list!
## THAR (ThAr-ThAr)
datadir = basedir/'thar'
anadir = basedir/'reduc'
if band=='h':
rawtag='IRDAD000'
elif band=='y':
rawtag='IRDBD000'
#wavelength calibration
thar=irdstream.Stream2D("thar",datadir,anadir,rawtag=rawtag,fitsid=list(range(14632,14732)))
thar.trace = trace_mmf
thar.clean_pattern(trace_mask=trace_mask,extin='', extout='_cp', hotpix_mask=hotpix_mask)
thar.calibrate_wavelength()
fitsid: [14632, 14633, 14634, 14635, 14636, 14637, 14638, 14639, 14640, 14641, 14642, 14643, 14644, 14645, 14646, 14647, 14648, 14649, 14650, 14651, 14652, 14653, 14654, 14655, 14656, 14657, 14658, 14659, 14660, 14661, 14662, 14663, 14664, 14665, 14666, 14667, 14668, 14669, 14670, 14671, 14672, 14673, 14674, 14675, 14676, 14677, 14678, 14679, 14680, 14681, 14682, 14683, 14684, 14685, 14686, 14687, 14688, 14689, 14690, 14691, 14692, 14693, 14694, 14695, 14696, 14697, 14698, 14699, 14700, 14701, 14702, 14703, 14704, 14705, 14706, 14707, 14708, 14709, 14710, 14711, 14712, 14713, 14714, 14715, 14716, 14717, 14718, 14719, 14720, 14721, 14722, 14723, 14724, 14725, 14726, 14727, 14728, 14729, 14730, 14731]
Skipped 100 files because they already exists.
0it [00:00, ?it/s]
median combine: _cp
100%|█████████████████████████████████████████████████████████████████████████████████████████████████████████████| 100/100 [00:00<00:00, 1310.82it/s]
/Users/yuikasagi/miniforge3/envs/py39_pip/lib/python3.9/site-packages/numpy/lib/nanfunctions.py:1218: RuntimeWarning: All-NaN slice encountered
r, k = function_base._ureduce(a, func=_nanmedian, axis=axis, out=out,
To reduce the fringe appearing in a spectrum, a process like
[`hdsis_ecf''](https://github.com/chimari/hds_iraf) for HDS/Subaru data is applied. In the preparation of this process, we create the normalized flat by using
apnormalize.`
## FLAT
if mmf=='mmf2':
## FLAT_STAR
datadir = basedir/'flat/'
anadir = basedir/'reduc/'
flat_star=irdstream.Stream2D("flat_star",datadir,anadir)
flat_star.fitsid=list(range(41804,41904,2))
flat_star.trace = trace_mmf
flat_star.band=band
if band == 'h' and flat_star.fitsid[0]%2==0:
flat_star.fitsid_increment()
flat_star.clean_pattern(trace_mask=trace_mask,extin='', extout='_cp', hotpix_mask=hotpix_mask)
flat_star.imcomb = True # median combine
flat_star.flatten(hotpix_mask=hotpix_mask)
df_flatn = flat_star.apnormalize()
elif mmf=='mmf1':
flat_comb.trace = trace_mmf
flat_comb.clean_pattern(trace_mask=trace_mask,extin='', extout='_cp', hotpix_mask=hotpix_mask)
flat_comb.imcomb = True # median combine
flat_comb.flatten(hotpix_mask=hotpix_mask)
df_flatn = flat_comb.apnormalize()
No fitsid yet.
Skipped 50 files because they already exists.
0it [00:00, ?it/s]
continuum is fitted with order_fit = 23.
median combine: _cp
100%|████████████████████████████████████████████████████████████████████████████████████████████████████████████████| 50/50 [00:00<00:00, 408.17it/s]
/Users/yuikasagi/miniforge3/envs/py39_pip/lib/python3.9/site-packages/numpy/lib/nanfunctions.py:1218: RuntimeWarning: All-NaN slice encountered
r, k = function_base._ureduce(a, func=_nanmedian, axis=axis, out=out,
100%|█████████████████████████████████████████████████████████████████████████████████████████████████████████████████| 21/21 [00:05<00:00, 3.53it/s]
The preparation of calibration data is now complete!
Extract target 1D spectrum
From here, we will extract target spectrum.
#--------FOR TARGET--------#
datadir = basedir/'target/'
anadir = basedir/'reduc/'
target = irdstream.Stream2D(
'targets', datadir, anadir, fitsid=[41510])
if band=='h' and target.fitsid[0]%2==0:
target.fitsid_increment() # when you use H-band
target.info = True # show detailed info
target.trace = trace_mmf
fitsid: [41510]
Remove noise pattern on detector by clean_pattern
.
# clean pattern
target.clean_pattern(trace_mask=trace_mask,extin='', extout='_cp', hotpix_mask=hotpix_mask)
clean_pattern: output extension= _cp
Ignore IRDA00041511.fits -> IRDA00041511_cp.fits
0it [00:00, ?it/s]
In apext_flatfield
, each order will be extracted with the flat
fielding. The option of hotpix_mask
determines whether hotpixels are
masked or not.
# flatten
target.apext_flatfield(df_flatn,hotpix_mask=hotpix_mask)
Ignore IRDA00041511_cp.fits -> IRDA00041511_flnhp_m2.fits
0it [00:00, ?it/s]
If there is set any hotpixel mask, *IRDA000?????_flnhp.fits* will be
created in anadir
.
Or, if hotpix_mask=None
(default), *IRDA000?????_fln.fits* will be
created in anadir
.
Assign wavelength to extracted spectrum.
The option extin
determines whether using the hotpixel masked and
flat fielded spectrum (extin='_flnhp'
) or not (extin='fln'
(default)).
# assign reference spectra & resample
target.dispcor(master_path=thar.anadir,extin='_flnhp')
dispcor: output spectrum= w41511_m2.dat
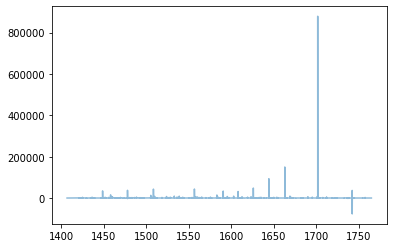
*w?????_m?.dat* will be created, whose data format is
$1: Wavelength [nm]
, $2: Order
, $3: Counts
.
*w?????_m?.dat* reflects the blaze function, so we want to “normalize” the spectra.
The blaze function of each order are created by applying the same aperture extract procedure to Flat image.
# blaze function
if mmf=='mmf2':
flat_star.apext_flatfield(df_flatn,hotpix_mask=hotpix_mask)
flat_star.dispcor(master_path=thar.anadir)
elif mmf=='mmf1':
flat_comb.apext_flatfield(df_flatn,hotpix_mask=hotpix_mask)
flat_comb.dispcor(master_path=thar.anadir)
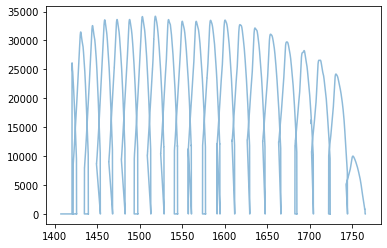
Flat spectrum is now extracted, and wflat_h_m?.dat is created.
Then, fit the continuum of the flat spectrum as blaze function and devide target spectrum by them.
# combine & normalize
if mmf=='mmf2':
target.normalize1D(master_path=flat_star.anadir,skipLFC=skipLFC)
elif mmf=='mmf1':
target.normalize1D(master_path=flat_comb.anadir,skipLFC=skipLFC)
Readout Noise is : 7.9305032251889385
/Users/yuikasagi/miniforge3/envs/py39_pip/lib/python3.9/site-packages/pandas/core/arraylike.py:397: RuntimeWarning: invalid value encountered in sqrt
result = getattr(ufunc, method)(*inputs, **kwargs)
/Users/yuikasagi/miniforge3/envs/py39_pip/lib/python3.9/site-packages/pandas/core/arraylike.py:397: RuntimeWarning: invalid value encountered in sqrt
result = getattr(ufunc, method)(*inputs, **kwargs)
normalize1D: output normalized 1D spectrum= nw41511_m2.dat
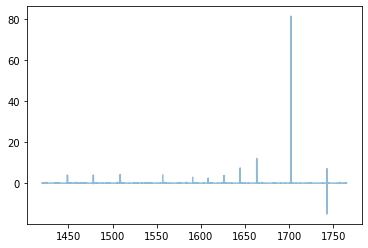
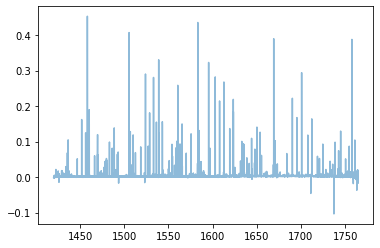
After normalize1D
, normalized spectrum (*nw?????_m?.dat*) will be
created.
The data format is $1: Wavelength [nm]
, $2: Order
,
$3: Counts
, $4: S/N
, $5: Uncertainties
.
In addition, there will be *ncw?????_m?.dat*, which is the order combined spectrum.
There are overlapping wavelengths at the edge of order, so we “normalize” the spectrum after summing up the flux of that regions to higher signal-to-noise ratio.
The data format is $1: Wavelength [nm]
, $2: Counts
, $3: S/N
,
$4: Uncertainties
.