Ackerman and Marley Cloud Modelο
Here, we try to compute a cloud opacity using Ackerman and Marley Model.
Although atmphys.AmpAmcloud
can easily compute the parameters of the
AM model, we here try to run the methods one by one. We consider
enstatite (MgSiO3).
import jax.numpy as jnp
import matplotlib.pyplot as plt
import numpy as np
Sets a simple atmopheric model. We need the density of atmosphere.
from exojax.utils.constants import kB, m_u
from exojax.atm.atmprof import pressure_layer_logspace
from exojax.utils.astrofunc import gravity_jupiter
Parr, dParr, k = pressure_layer_logspace(log_pressure_top=-4., log_pressure_btm=6.0, nlayer=100)
alpha = 0.097
T0 = 1200.
Tarr = T0 * (Parr)**alpha
mu = 2.3 # mean molecular weight
R = kB / (mu * m_u)
rho = Parr / (R * Tarr)
gravity = gravity_jupiter(1.0, 1.0)
The solar abundance can be obtained using utils.zsol.nsol. Here, we assume a maximum mol Mixing Ratio for MgSiO3 and Fe from solar abundance.
from exojax.utils.zsol import nsol
n = nsol() #solar abundance
MolMR_enstatite = np.min([n["Mg"], n["Si"], n["O"] / 3])
MolMR_Fe = n["Fe"]
Vapor saturation pressures can be obtained using atm.psat
from exojax.atm.psat import psat_enstatite_AM01
P_enstatite = psat_enstatite_AM01(Tarr)
Computes a cloud base pressure.
from exojax.atm.amclouds import compute_cloud_base_pressure
Pbase_enstatite = compute_cloud_base_pressure(Parr, P_enstatite, MolMR_enstatite)
The cloud base is located at the intersection of a TP profile and the vapor saturation puressure devided by VMR.
plt.plot(Tarr, Parr, color="black", ls="dashed", label="T - P profile")
plt.plot(Tarr,
P_enstatite / MolMR_enstatite,
label="$P_{sat}/\\xi$ (enstatite)",
color="gray")
plt.axhline(Pbase_enstatite, color="gray", alpha=0.7, ls="dotted")
plt.text(500, Pbase_enstatite * 0.8, "cloud base (enstatite)", color="gray")
plt.yscale("log")
plt.ylim(1.e-4, 1.e5)
plt.xlim(0, 3000)
plt.gca().invert_yaxis()
plt.legend()
plt.xlabel("Temperature (K)")
plt.ylabel("Pressure (bar)")
plt.savefig("pbase.pdf", bbox_inches="tight", pad_inches=0.0)
plt.savefig("pbase.png", bbox_inches="tight", pad_inches=0.0)
plt.show()
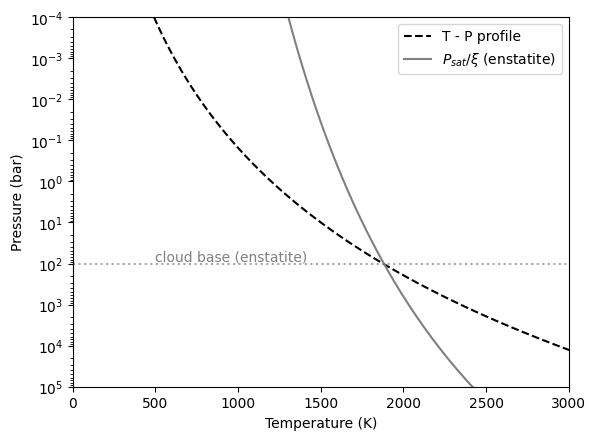
Compute Mass Mixing Ratio (MMRs) of clouds. In this block, we first convert mol mixing ratio of condensates to MMR, then computes a cloud profile.
from exojax.atm.amclouds import mixing_ratio_cloud_profile
from exojax.spec.molinfo import molmass_isotope
from exojax.atm.atmconvert import vmr_to_mmr
fsed = 3.
muc_enstatite = molmass_isotope("MgSiO3")
MMRbase_enstatite = vmr_to_mmr(MolMR_enstatite, muc_enstatite,mu)
MMRc_enstatite = mixing_ratio_cloud_profile(Parr, Pbase_enstatite, fsed, MMRbase_enstatite)
['H2O', 'CO2', 'O3', 'N2O', 'CO', 'CH4', 'O2', 'NO', 'SO2', 'NO2', 'NH3', 'HNO3', 'OH', 'HF', 'HCl', 'HBr', 'HI', 'ClO', 'OCS', 'H2CO', 'HOCl', 'N2', 'HCN', 'CH3Cl', 'H2O2', 'C2H2', 'C2H6', 'PH3', 'COF2', 'SF6', 'H2S', 'HCOOH', 'HO2', 'O', 'ClONO2', 'NO+', 'HOBr', 'C2H4', 'CH3OH', 'CH3Br', 'CH3CN', 'CF4', 'C4H2', 'HC3N', 'H2', 'CS', 'SO3', 'C2N2', 'COCl2', 'SO', 'CH3F', 'GeH4', 'CS2', 'CH3I', 'NF3']
/home/kawahara/exojax/src/exojax/spec/molinfo.py:64: UserWarning: db_HIT is set as True, but the molecular name 'MgSiO3' does not exist in the HITRAN database. So set db_HIT as False. For reference, all the available molecules in the HITRAN database are as follows:
warnings.warn(warn_msg, UserWarning)
The followings are the base pressures for enstatite and Fe.
print(Pbase_enstatite)
104.62701
Here is the MMR distribution.
plt.figure()
plt.gca().get_xaxis().get_major_formatter().set_powerlimits([-3, 3])
plt.plot(MMRc_enstatite, Parr, color="gray", label="MMR (enstatite)")
plt.yscale("log")
#plt.ylim(1.e-7, 10000)
plt.gca().invert_yaxis()
plt.legend()
plt.xlabel("Mass Mixing Ratio (clouds)")
plt.ylabel("Pressure (bar)")
plt.savefig("mmrcloud.pdf", bbox_inches="tight", pad_inches=0.0)
plt.savefig("mmrcloud.png", bbox_inches="tight", pad_inches=0.0)
plt.show()
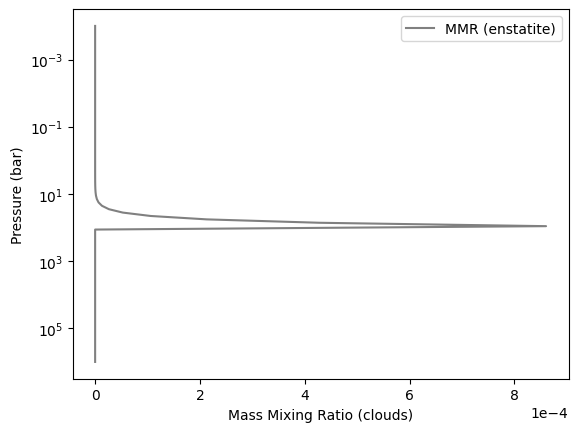
Computes dynamic viscosity in H2 atmosphere (cm/g/s)
from exojax.atm.viscosity import eta_Rosner, calc_vfactor
T = np.logspace(np.log10(1000), np.log10(2000))
vfactor, Tr = calc_vfactor("H2")
eta = eta_Rosner(T, vfactor)
plt.plot(T, eta)
plt.xscale("log")
plt.yscale("log")
plt.xlabel("Temperature (K)")
plt.ylabel("Dynamic viscosity (cm/g/s)")
plt.show()
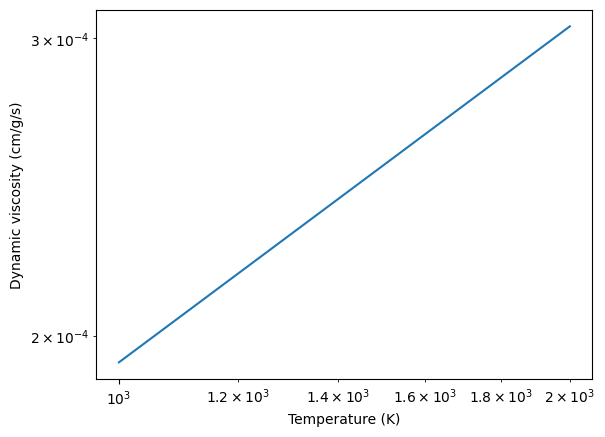
The pressure scale height can be computed using atm.atmprof.Hatm.
from exojax.atm.atmprof import pressure_scale_height
T = 1000 #K
print("scale height=", pressure_scale_height(1.e5, T, mu), "cm")
scale height= 361498.2549385839 cm
We need the substance density of condensates.
from exojax.atm.condensate import condensate_substance_density, name2formula
deltac_enstatite = condensate_substance_density[name2formula["enstatite"]]
Letβs compute the terminal velocity. We can compute the terminal velocity of cloud particle using atm.vterm.vf. vmap is again applied to vf.
from exojax.atm.viscosity import calc_vfactor, eta_Rosner
from exojax.atm.vterm import terminal_velocity
from jax import vmap
vfactor, trange = calc_vfactor(atm="H2")
rarr = jnp.logspace(-6, -4, 2000) #cm
drho = deltac_enstatite - rho
eta_fid = eta_Rosner(Tarr, vfactor)
g = 1.e5
vf_vmap = vmap(terminal_velocity, (None, None, 0, 0, 0))
vfs = vf_vmap(rarr, g, eta_fid, drho, rho)
Kzz/L will be used to calibrate \(r_w\). following Ackerman and Marley 2001
#sigmag:sigmag parameter (geometric standard deviation) in the lognormal distribution of condensate size, defined by (9) in AM01, must be sigmag > 1
Kzz = 1.e5 #cm2/s
sigmag = 2.0 # > 1
alphav = 1.3
L = pressure_scale_height(g, 1500, mu)
Kzz/L
0.18441767216274083
for i in range(0, len(Tarr)):
plt.plot(rarr, vfs[i, :], alpha=0.2, color="gray")
plt.xscale("log")
plt.yscale("log")
plt.axhline(Kzz / L, label="Kzz/H", color="C2", ls="dotted")
plt.ylabel("stokes terminal velocity (cm/s)")
plt.xlabel("condensate size (cm)")
Text(0.5, 0, 'condensate size (cm)')
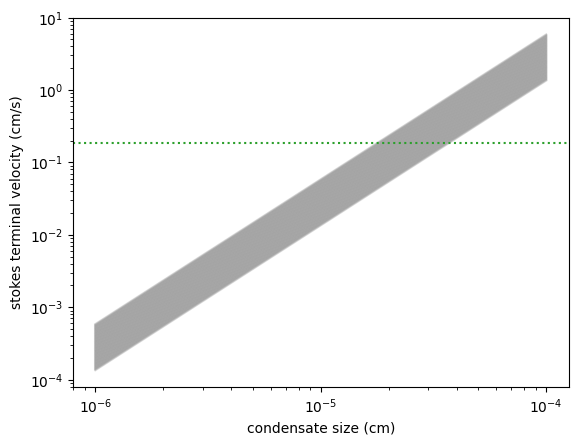
Find the intersection.
from exojax.atm.amclouds import find_rw
vfind_rw = vmap(find_rw, (None, 0, None), 0)
rw = vfind_rw(rarr, vfs, Kzz / L)
Then, \(r_g\) can be computed from \(r_w\) and other quantities.
from exojax.atm.amclouds import get_rg
rg = get_rg(rw, fsed, alphav, sigmag)
plt.plot(rg * 1.e4, Parr, label="$r=r_g$", color="black")
plt.plot(rw * 1.e4, Parr, ls="dashed", label="$r=r_w$", color="black")
#plt.ylim(1.e-7, 10000)
plt.xlabel("$r$ (micron)")
plt.ylabel("Pressure (bar)")
plt.yscale("log")
plt.savefig("rgrw.png")
plt.legend()
<matplotlib.legend.Legend at 0x74b83ea63d00>
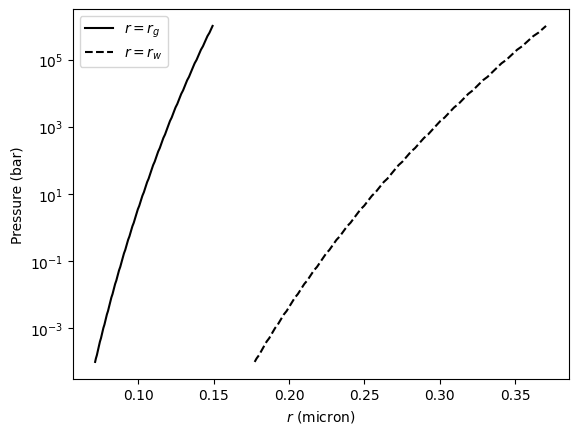
These processes can be reprodced using AmpAmcloud
, which uses
PdbCloud
as one of the input arguments. Here, we show an example:
from exojax.atm.atmphys import AmpAmcloud
from exojax.spec.pardb import PdbCloud
pdb_enstatite = PdbCloud("MgSiO3")
pdb_Fe = PdbCloud("Fe")
amp = AmpAmcloud(pdb_enstatite,bkgatm="H2")
rg, MMRc = amp.calc_ammodel(Parr,Tarr,mu,MMRc_enstatite,gravity,fsed,sigmag,Kzz,MMRbase_enstatite,alphav=alphav)
.database/particulates/virga/virga.zip exists. Remove it if you wanna re-download and unzip.
Refractive index file found: .database/particulates/virga/MgSiO3.refrind
Miegrid file does not exist at .database/particulates/virga/miegrid_lognorm_MgSiO3.mg.npz
Generate miegrid file using pdb.generate_miegrid if you use Mie scattering
.database/particulates/virga/virga.zip exists. Remove it if you wanna re-download and unzip.
Refractive index file found: .database/particulates/virga/Fe.refrind
Miegrid file does not exist at .database/particulates/virga/miegrid_lognorm_Fe.mg.npz
Generate miegrid file using pdb.generate_miegrid if you use Mie scattering
from exojax.spec.layeropacity import layer_optical_depth_cloudgeo
dtau_enstatite = layer_optical_depth_cloudgeo(Parr, deltac_enstatite, MMRc_enstatite, rg, sigmag, g)
The Mie scattering can be computed using OpaMie
.
from exojax.utils.grids import wavenumber_grid
from exojax.spec.unitconvert import wav2nu
N = 1000
wavelength_start = 5000.0 # AA
wavelength_end = 15000.0 # AA
margin = 10 # cm-1
nus_start = wav2nu(wavelength_end, unit="AA") - margin
nus_end = wav2nu(wavelength_start, unit="AA") + margin
nugrid, wav, res = wavenumber_grid(nus_start, nus_end, N, xsmode="lpf", unit="cm-1")
from exojax.spec.opacont import OpaMie
opa_enstatite = OpaMie(pdb_enstatite, nugrid)
rg = 1.0e-4 # 0.1um
# beta0, betasct, g = opa.mieparams_vector(rg,sigmag) # if you've already generated miegrid
beta0, betasct, g = opa_enstatite.mieparams_vector_direct_from_pymiescatt(
rg, sigmag
) # uses direct computation of Mie params using PyMieScatt
from exojax.spec.layeropacity import layer_optical_depth_clouds_lognormal
dtau_enstatite_mie = layer_optical_depth_clouds_lognormal(
dParr, beta0, deltac_enstatite, MMRc_enstatite, rg, sigmag, gravity
)
xsmode = lpf xsmode assumes ESLOG in wavenumber space: mode=lpf ====================================================================== The wavenumber grid should be in ascending order. The users can specify the order of the wavelength grid by themselves. Your wavelength grid is in * descending * order ======================================================================
100%|ββββββββββ| 63/63 [00:17<00:00, 3.57it/s]
The difference of the geometric approximation and Mie scattering is a bit.
fig = plt.figure()
ax=fig.add_subplot(111)
plt.plot(dtau_enstatite, Parr, color="C1", ls="dashed", label="geometric approximation")
plt.plot(np.median(dtau_enstatite_mie,axis=1), Parr, color="C3", label="Mie",alpha=0.5,lw=2)
plt.legend()
plt.yscale("log")
plt.xlabel("$d\\tau$")
plt.ylabel("Pressure (bar)")
#plt.xscale("log")
plt.gca().invert_yaxis()
plt.show()
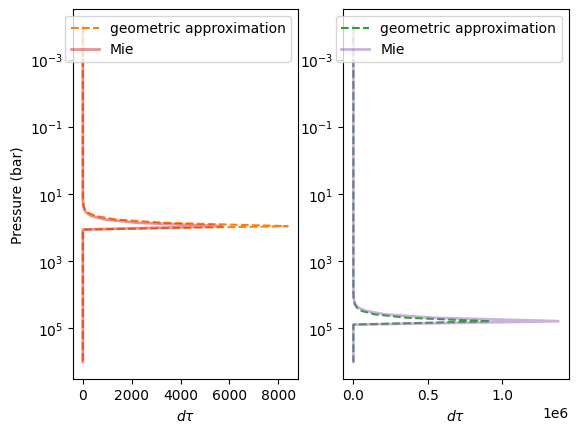
Letβs compare with CIA
#CIA
from exojax.spec import contdb
cdbH2H2 = contdb.CdbCIA('.database/H2-H2_2011.cia', nugrid)
H2-H2
from exojax.spec.layeropacity import layer_optical_depth_CIA
from exojax.atm.atmconvert import mmr_to_vmr
mmrH2 = 0.74
molmassH2 = molmass_isotope("H2")
vmrH2 = mmr_to_vmr(mmrH2, mu, molmassH2)
dtaucH2H2 = layer_optical_depth_CIA(
nugrid,
Tarr,
Parr,
dParr,
vmrH2,
vmrH2,
mu,
gravity,
cdbH2H2.nucia,
cdbH2H2.tcia,
cdbH2H2.logac,
)
dtau = dtaucH2H2 + dtau_enstatite_mie
from exojax.plot.atmplot import plotcf
plotcf(nugrid, dtau, Tarr, Parr, dParr, unit="AA")
plt.show()

from exojax.plot.atmplot import plotcf
plotcf(nugrid, dtaucH2H2, Tarr, Parr, dParr, unit="AA")
plt.show()

from exojax.plot.atmplot import plotcf
plotcf(nugrid,
dtau_enstatite_mie,
Tarr,
Parr,
dParr,
unit="AA")
plt.show()

from exojax.spec import planck
from exojax.spec.rtransfer import rtrun_emis_pureabs_fbased2st as rtrun
#from exojax.spec.rtransfer import rtrun_emis_pureabs_ibased as rtrun
sourcef = planck.piBarr(Tarr, nugrid)
F0 = rtrun(dtau, sourcef)
F0CIA = rtrun(dtaucH2H2, sourcef)
F0cl = rtrun(dtau_enstatite[:, None] + np.zeros_like(dtaucH2H2), sourcef)
In this case, the effect of clouds and CIA are comparable with each other
plt.plot(wav, F0, label="Clouds+CIA")
plt.plot(wav, F0CIA, label="CIA only", ls="dashed")
plt.plot(wav, F0cl, label="Clouds only", ls="dotted")
plt.xlabel("wavelenght AA")
plt.legend()
plt.show()
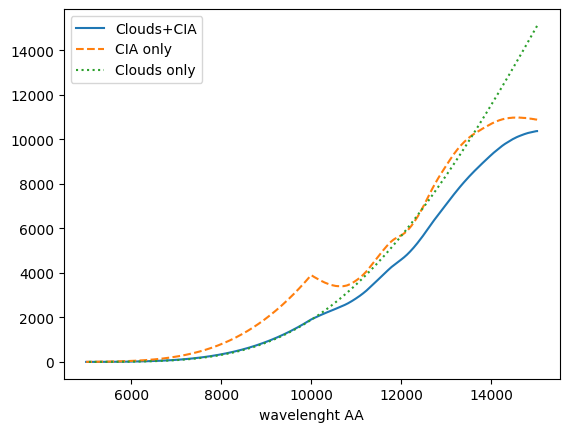